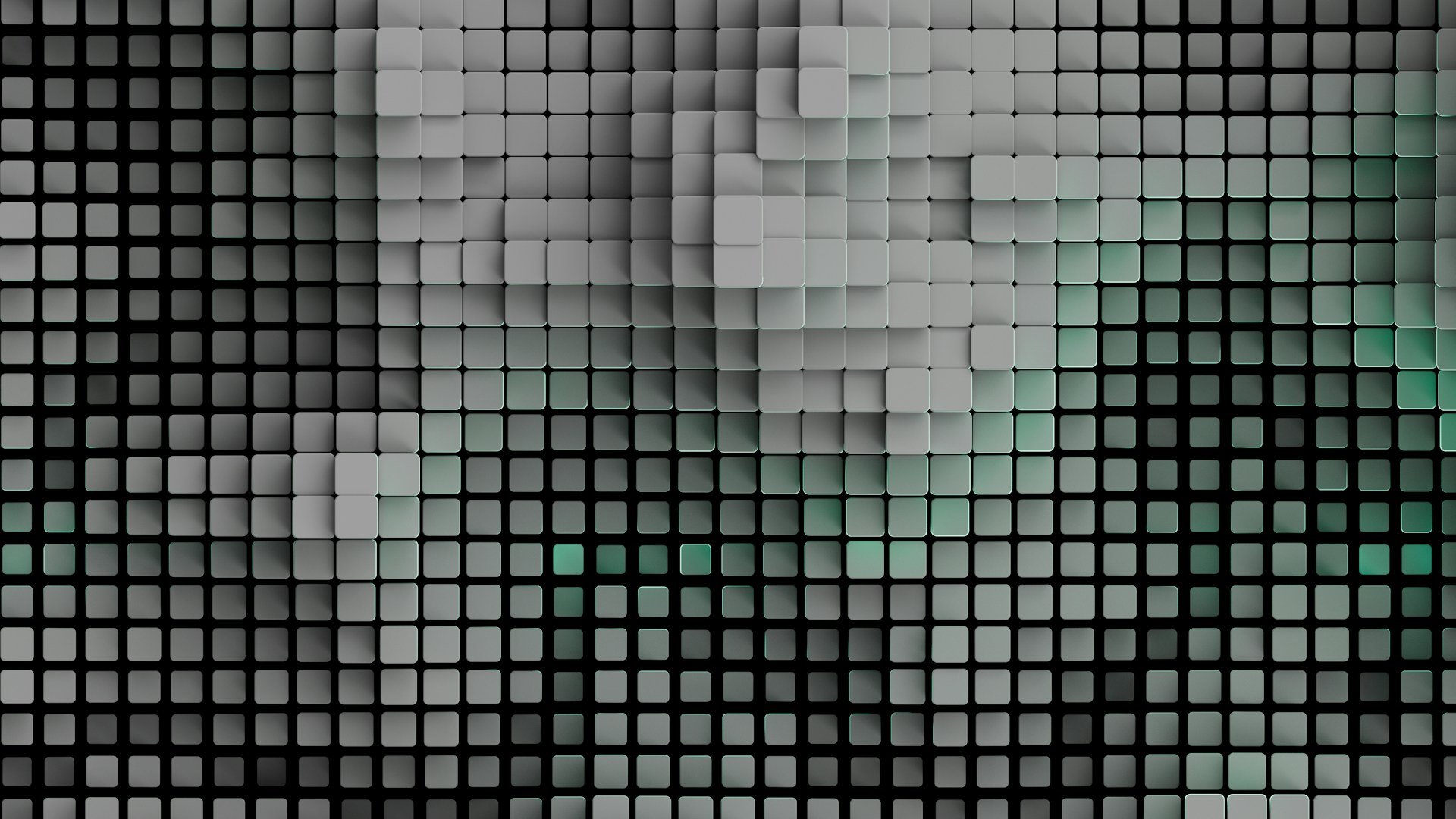
Data Quality & Enrichment
Data quality refers to the condition of a set of data, which is crucial for ensuring its reliability and usefulness for decision-making processes. High-quality data is characterised by its accuracy, consistency, completeness, timeliness, and relevance. Accurate data accurately represents real-world conditions, while consistency ensures uniformity across different datasets and platforms.
Completeness means that all necessary data points are present, leaving no gaps that could lead to incorrect conclusions. Timeliness refers to the data being up-to-date and available when needed, and relevance ensures the data is applicable to the specific context or decision at hand.
Maintaining high data quality is essential for businesses as it enhances the credibility of analytics, supports effective strategy development, and ensures compliance with regulatory requirements. Poor data quality can lead to misguided decisions, operational inefficiencies, and loss of customer trust, making it imperative for organisations to implement robust data governance and quality assurance practices.
Key Focus Areas:
Profiling
Deduplication & Cleansing
Refinement
Predictive & Forecast Analysis
Ontology & Product Enrichment
Feed Management
Governance & Security
Storage
Connectivity & Integration Routes
MDM
Metadata
Toolsets:
GA
Mixpanel
Talend
Snowflake
Data Refinery
Store Hero
Open Refine
Others coming soon
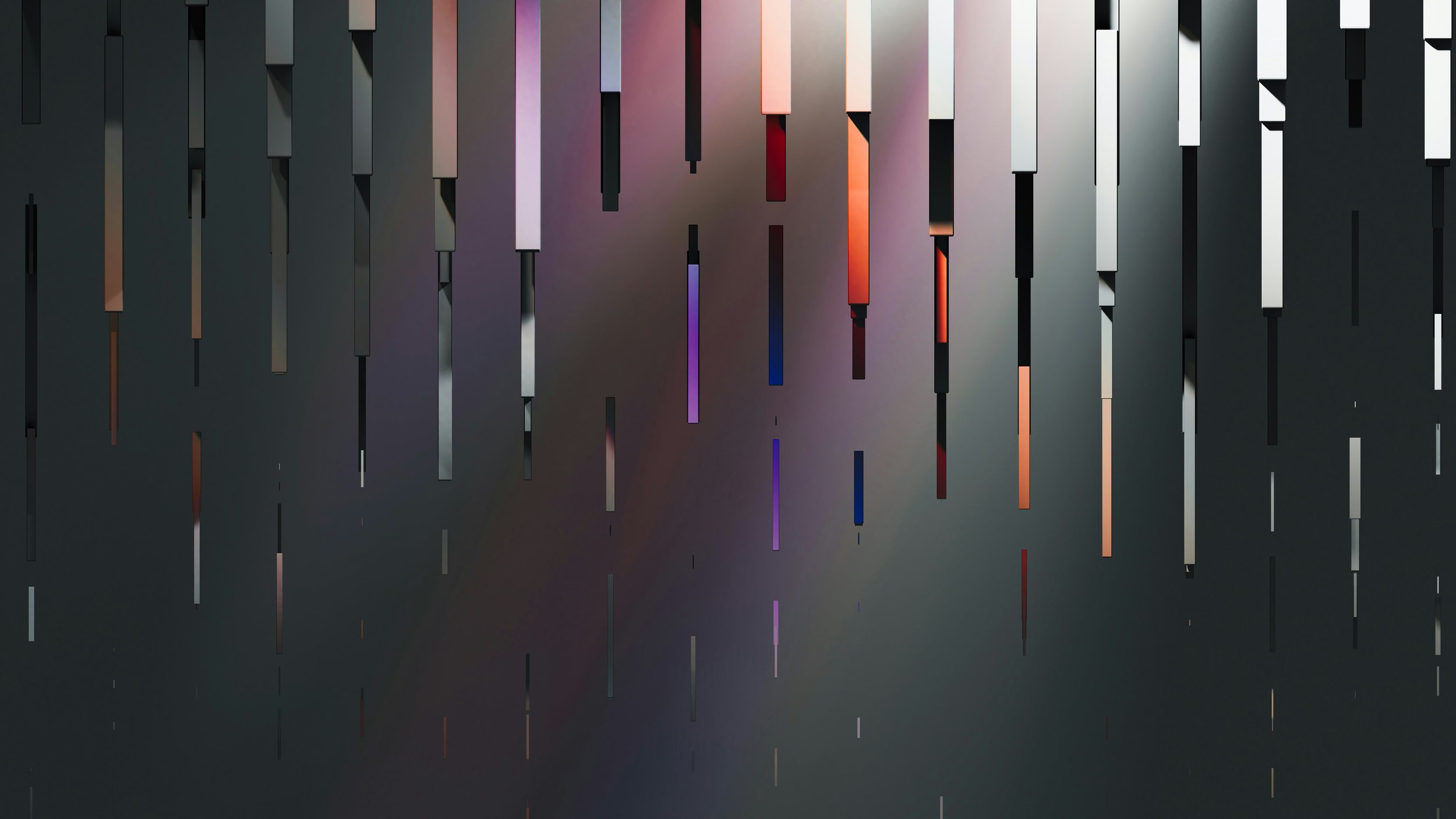